Unlocking the Future: The Essential Role of Video Annotation Tools for Machine Learning
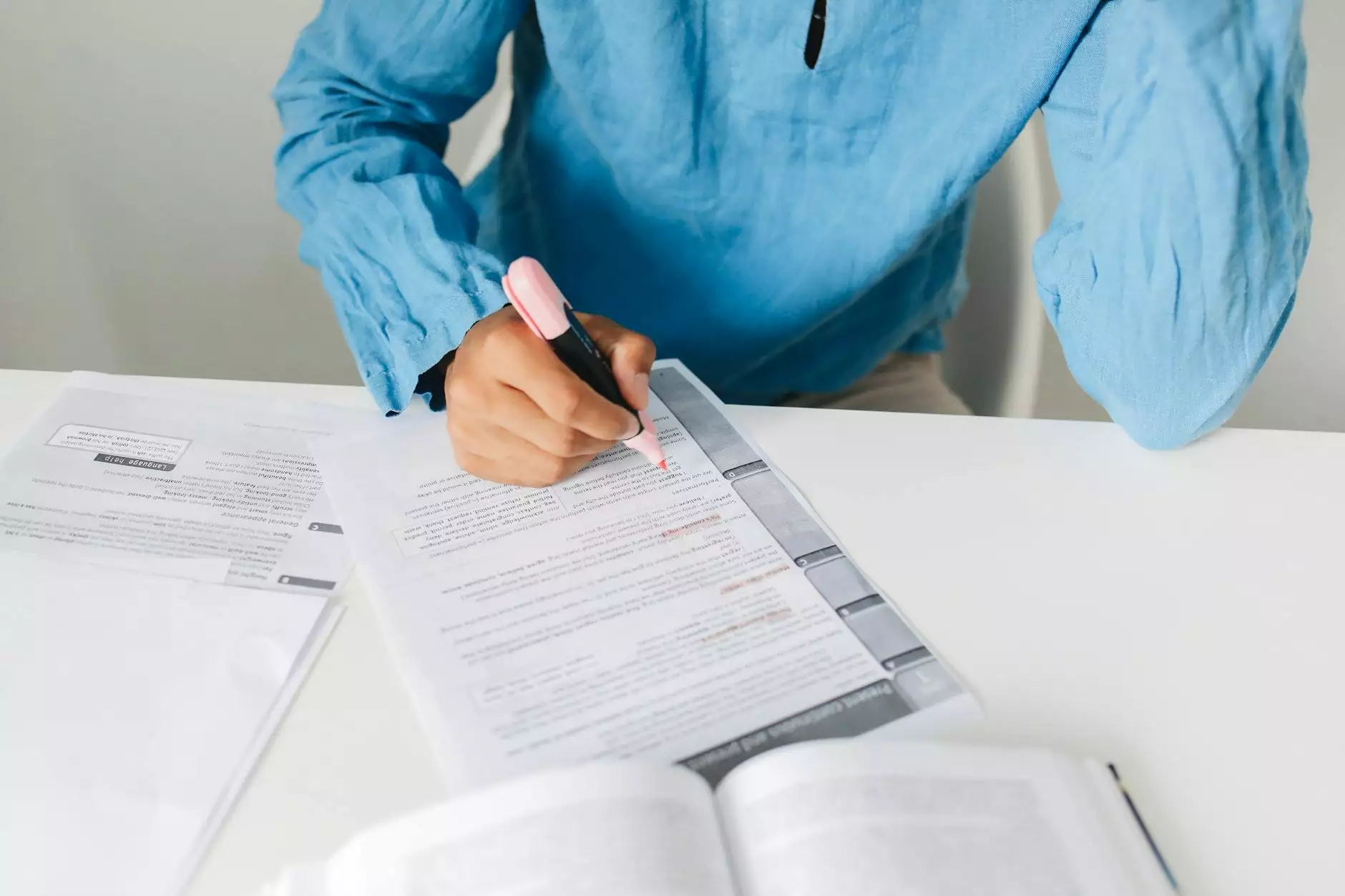
Introduction to Video Annotation in Machine Learning
In the world of artificial intelligence (AI), the process of data labeling is crucial for training models and algorithms that power sophisticated applications. Among various data types, video data poses unique challenges and opportunities. This brings us to an essential component of machine learning: the video annotation tool for machine learning.
Video annotation tools help in converting raw video content into structured data, allowing machine learning models to learn from visual inputs. In this article, we'll explore the functionalities, benefits, and innovations surrounding video annotation tools, focusing on their impact in driving advancements in AI.
Understanding Video Annotation Tools
Video annotation tools are software applications specifically designed to facilitate the process of labeling video content. These tools enable users to tag, segment, and categorize different elements within videos, which is essential for applications ranging from autonomous vehicles to security systems, and beyond.
The process of video annotation typically includes:
- Object Detection: Identifying and labeling objects within a frame.
- Segmentation: Dividing the video into segments based on user requirements.
- Tracking: Following objects as they move across frames.
- Classification: Categorizing scenes or objects based on defined criteria.
The Importance of Video Annotation for Machine Learning
Video data is abundant, particularly with the growth of social media and surveillance technologies. However, machine learning algorithms require meticulously labeled data to function effectively. This is where video annotation tools come in. Their importance cannot be overstated:
- Enhanced Learning: Labeled video data improves the learning accuracy of AI algorithms, allowing them to make better predictions and decisions.
- Scalability: Automated video annotation tools can process large datasets efficiently, making it scalable for enterprises.
- Cost-Effectiveness: By utilizing these tools, businesses can significantly reduce the costs associated with manual annotation.
- Increased Precision: Advanced tools offer features like frame-by-frame annotation, ensuring high levels of accuracy in data labeling.
Key Features of a Video Annotation Tool
When selecting a video annotation tool for machine learning, it’s essential to look for several key features that can enhance usability and efficiency:
- User-Friendly Interface: A tool with an intuitive interface can minimize the learning curve for users.
- Real-Time Collaboration: Collaboration features allow multiple users to work on video annotation simultaneously, improving productivity.
- Automation Capabilities: Incorporating AI to assist in labeling can drastically speed up the annotation process.
- Robust Export Options: Ability to export data in various formats suited for different machine learning frameworks.
- Quality Control Features: Ensure accuracy in annotations through review systems or automated checks.
Applications of Video Annotation in Machine Learning
Various industries are leveraging video annotation tools to boost their machine learning applications. Some prominent applications include:
Autonomous Vehicles
Video annotation is critical in developing self-driving cars, as it aids in object detection, lane detection, and identifying various traffic signs and signals.
Healthcare Monitoring
In healthcare, video surveillance of patients can help in monitoring patient behavior, detecting falls, or other emergency situations, all of which require precise video annotation for effective outcome analysis.
Security and Surveillance
Enhancements in security systems utilize video annotation for identifying suspicious behaviors through video analysis, thereby improving the safety and security infrastructure.
Sports Analytics
In sports, video annotation tools are used to analyze player movements, tactics, and overall performance, yielding insights that can help teams improve their strategies and results.
Choosing the Right Video Annotation Tool
Selecting a suitable video annotation tool for machine learning is crucial. Here are some factors to consider:
- Type of Annotation Needed: Ensure the tool meets your specific data labeling requirements, be it bounding boxes, segmentation, or tracking.
- Integration Capabilities: Check if the tool can integrate seamlessly with your existing machine learning framework or workflow.
- Budget and Pricing: Assess whether the tool's pricing structure aligns with your budgetary constraints, whether it’s a pay-per-use model or a subscription service.
- Customer Support: Evaluate the level of customer support offered, which can be vital during the implementation phase.
Future Trends in Video Annotation for Machine Learning
As technology continues to evolve, so does the domain of video annotation. A few trends shaping the future include:
Artificial Intelligence and Automation
The integration of AI will further automate the video annotation process, thereby allowing for quicker and more accurate annotations with minimal human intervention.
Edge Computing
The rise of edge computing will enable real-time video annotation on devices, reducing latency and allowing for immediate data processing, which is particularly beneficial in applications like autonomous driving.
Increased Accessibility Through Cloud Solutions
Cloud-based solutions are making video annotation tools more accessible, allowing users from different geographical locations to collaborate on projects seamlessly.
Conclusion
The emergence of video annotation tools represents a pivotal moment in the evolution of machine learning. As AI becomes increasingly integral to various sectors, the demand for high-quality annotated video data will continue to grow. Investing in a robust video annotation tool for machine learning is not just about keeping pace with technological trends; it's about positioning your business at the forefront of innovation.
At KeyLabs.ai, we understand the critical role that effective data annotation plays in machine learning success. We provide cutting-edge solutions tailored for your data annotation needs, ensuring your AI initiatives are powered by high-quality, precise labeled data.