Image Annotation for Machine Learning: Elevating Your Data Annotation Process
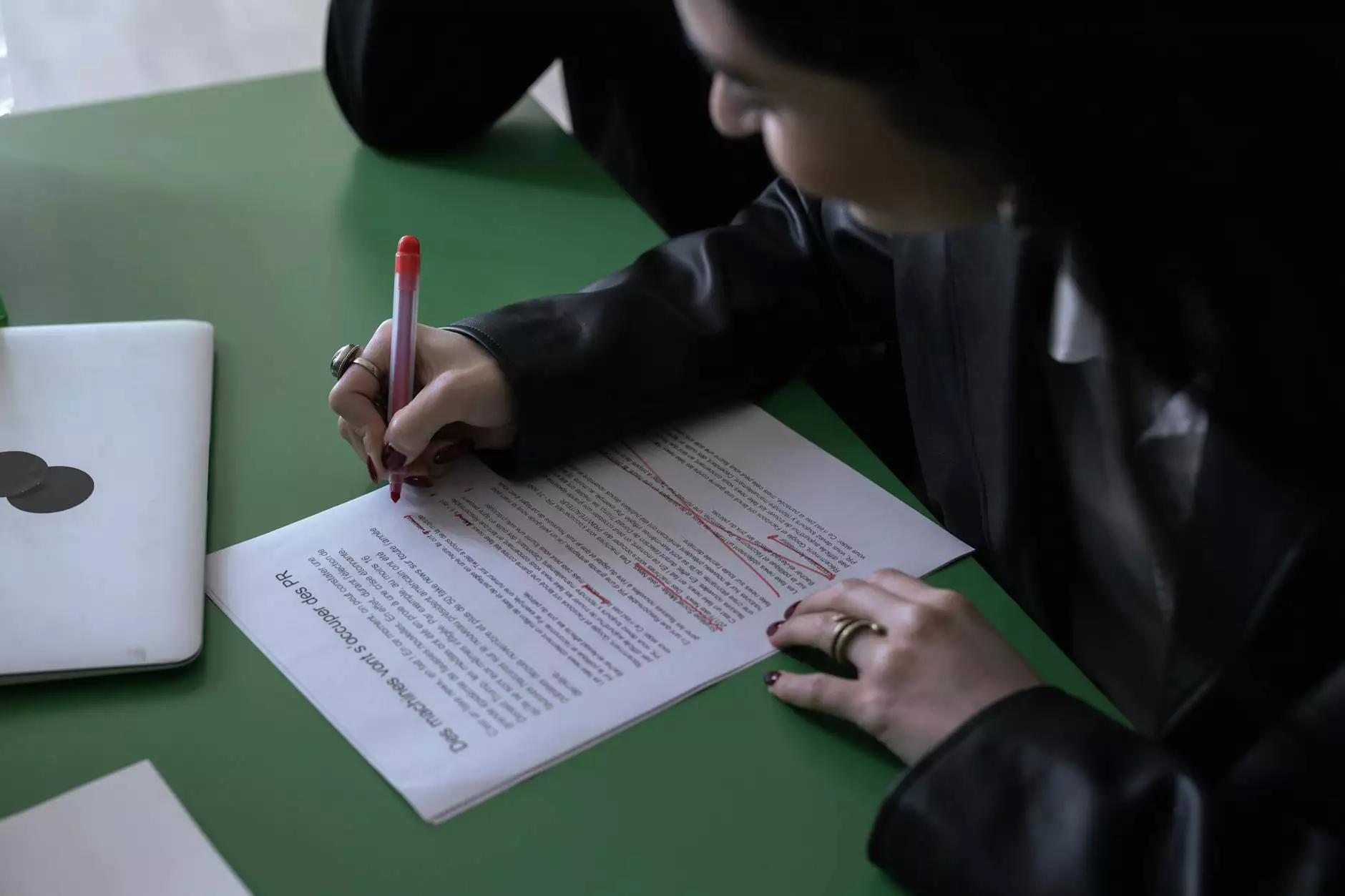
The Importance of Image Annotation in Machine Learning
When it comes to machine learning, especially in the field of computer vision, the accuracy and effectiveness of models depend heavily on the data used for training. One pivotal part of this process is image annotation, which is the cornerstone of creating high-quality datasets. This process involves labeling images with rich metadata, which allows algorithms to learn from structured data.
Without proper image annotation, machine learning models may struggle to accurately recognize patterns or objects within images, ultimately leading to poor performance in real-world applications. Thus, businesses and developers must prioritize employing comprehensive annotation strategies to enhance their machine learning initiatives.
Understanding Image Annotation Types
There are various types of image annotation techniques, each tailored to meet specific machine learning needs. These include:
- Bounding Box Annotation: This is commonly used to outline objects in images, making it easier for the model to detect and identify them. For example, in autonomous vehicles, bounding boxes can help identify pedestrians, other vehicles, and road signs.
- Polygon Annotation: This method provides a more precise outline of objects, which is essential for detailed tasks like segmenting irregular shapes in medical imaging.
- Semantic Segmentation: In this method, each pixel of the image is classified into a specific category. This is crucial for applications that require understanding the context within the whole image, such as in image editing or restoration.
- Keypoint Annotation: This technique focuses on specific points of interest within an image, commonly used in facial recognition and pose estimation.
Why Choose KeyLabs.ai for Image Annotation?
At KeyLabs.ai, we are dedicated to providing advanced data annotation tools and platforms that simplify the process of image annotation for machine learning. Our platform offers a user-friendly interface combined with powerful features that ensure your data annotation experience is efficient and effective.
- Scalability: We understand that businesses grow over time. Our platform supports scaling from small datasets to enterprise-level projects without compromising quality.
- Customization: Each project is unique, and we provide customizable annotation options tailored to your specific needs, ensuring that you receive the exact type of annotations required.
- Expert Annotators: Our team includes skilled annotators familiar with the latest machine learning trends, ensuring that your data is labeled accurately and consistently.
- Integrated Quality Assurance: We implement multiple quality checks throughout the annotation process to guarantee high accuracy and reliability in the data provided.
Best Practices in Image Annotation for Machine Learning
To maximize the impact of your image annotation efforts, consider the following best practices:
- Define Clear Objectives: Before initiating your annotation project, clearly outline what you aim to achieve. Understanding the end goal will guide your annotation style and technique.
- Train Annotators: Ensure that the individuals handling the annotation are well-trained and understand the guidelines. Regular training sessions can significantly enhance the quality of annotations.
- Utilize Advanced Tools: Leverage modern data annotation tools that incorporate machine learning algorithms to assist human annotators, thus increasing efficiency and consistency.
- Continuous Feedback Loop: Establish a feedback mechanism for annotators to refine their techniques continually, ensuring that high standards are maintained throughout the project.
Challenges in Image Annotation
While image annotation for machine learning is essential, it comes with its set of challenges. Understanding these can help in better planning and execution of your projects:
- Time-Consuming Processes: Depending on the volume of images and the complexity of annotations, this process can be very time-consuming without the right tools.
- Inconsistency in Annotations: Human errors can lead to variations in how images are labeled, which can adversely affect training data quality.
- High Costs: If choosing to manage in-house, the labor costs associated with training annotators and ongoing quality checks can add up significantly.
- Data Privacy Concerns: Handling sensitive information in images requires stringent security measures, which can complicate the annotation process.
The Future of Image Annotation in Machine Learning
As machine learning continues to evolve, so will the techniques and technologies surrounding image annotation. Emerging trends include:
- Automated Annotation Tools: The development of AI-driven tools that can automate a significant portion of the annotation process, drastically reducing the time and effort required.
- Collaborative Annotation Platforms: Tools that allow multiple users to annotate images simultaneously can increase efficiency and accuracy.
- Integration with Augmented Reality (AR): Future annotations may also involve AR techniques wherein real-time data overlay can support further visualization and contextual understanding.
- Focus on Data Privacy: Innovations in data security protocols will likely change how companies approach image annotation, ensuring that privacy regulations are upheld while still managing to annotate effectively.
Conclusion
In conclusion, image annotation for machine learning is a critical component in the development of effective machine learning models. With the right tools and strategies, businesses can enhance their data annotation efforts significantly. At KeyLabs.ai, we are committed to providing robust platforms and exemplary services that elevate your data annotation process, ensuring that your machine learning models achieve the highest possible accuracy and performance.
Embrace the future of machine learning with us, and let KeyLabs.ai be your partner in navigating the complexities of data annotation.